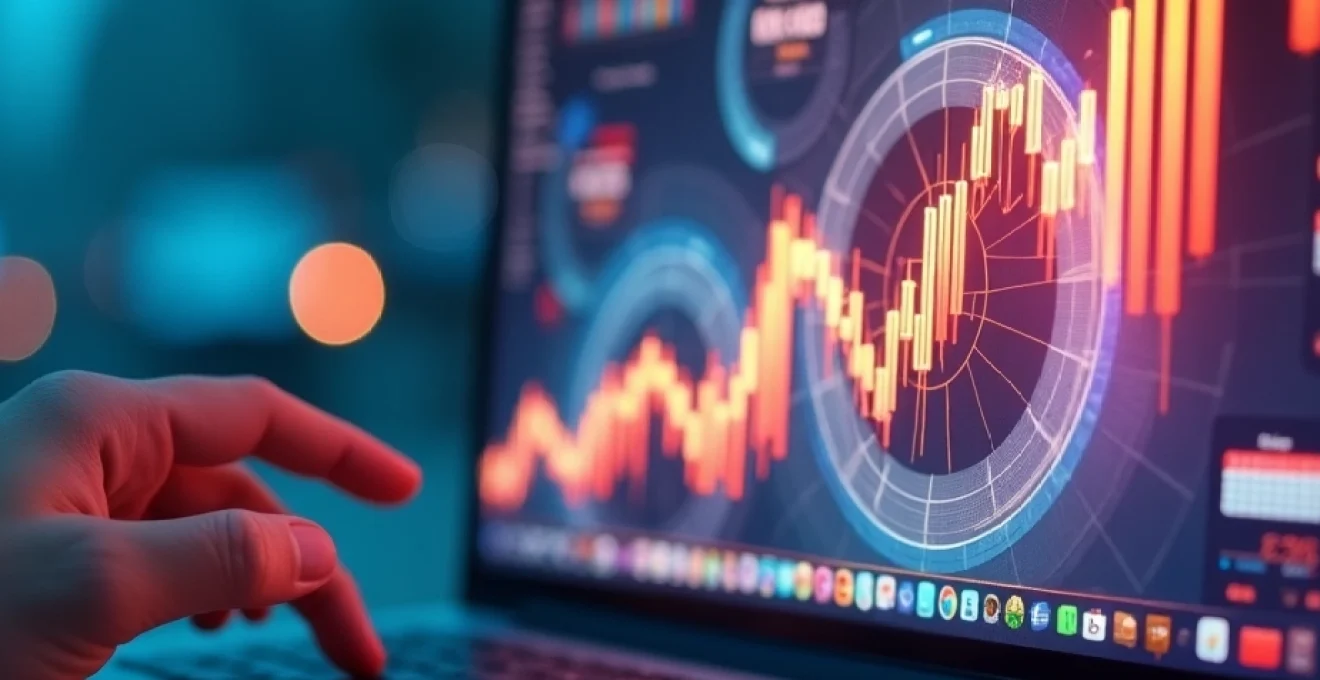
In today's digital landscape, targeted advertising has emerged as a powerful tool for businesses to connect with their audience and boost sales. By leveraging advanced technologies and data-driven strategies, companies can deliver personalized content that resonates with potential customers, increasing engagement and conversion rates. This approach not only enhances the user experience but also maximizes the return on investment for marketing efforts.
Targeted ads utilize sophisticated algorithms and consumer data to present relevant messages to specific segments of the population. By tailoring content to individual preferences and behaviors, businesses can create more meaningful connections with their audience, leading to higher click-through rates and ultimately, increased sales. Let's explore the various techniques and technologies that make targeted advertising an indispensable part of modern marketing strategies.
Behavioral targeting algorithms in digital advertising
At the heart of targeted advertising lies behavioral targeting algorithms. These complex systems analyze vast amounts of user data to predict consumer preferences and deliver highly relevant ads. By examining factors such as browsing history, purchase behavior, and online interactions, these algorithms can create detailed user profiles that inform ad targeting decisions.
One of the key advantages of behavioral targeting is its ability to reach consumers at different stages of the buying journey. For instance, a user who has recently searched for running shoes might be shown ads for athletic wear, while someone who has abandoned a shopping cart could receive a reminder about their unfinished purchase. This level of personalization significantly increases the likelihood of engagement and conversion.
Moreover, behavioral targeting allows advertisers to optimize their campaigns in real-time. By continuously analyzing user responses to different ad formats and messages, algorithms can refine their targeting criteria, ensuring that ads remain relevant and effective over time. This dynamic approach to advertising helps businesses stay agile in a rapidly changing market landscape.
Data-driven personalization techniques for ad content
Personalization is the cornerstone of effective targeted advertising. By tailoring ad content to individual users, businesses can create more engaging and memorable experiences that drive action. Data-driven personalization techniques leverage a wide range of information to craft ads that speak directly to the interests and needs of each consumer.
Machine learning models for user preference prediction
Machine learning models have revolutionized the way advertisers predict user preferences. These sophisticated algorithms can process enormous datasets to identify patterns and trends in consumer behavior. By analyzing factors such as past purchases, website interactions, and demographic information, machine learning models can make highly accurate predictions about a user's future interests and buying intentions.
For example, a machine learning model might determine that users who frequently browse travel websites and have recently searched for sunscreen are likely planning a beach vacation. Armed with this insight, advertisers can serve targeted ads for related products such as swimwear, beach accessories, or travel insurance. This level of precision in ad targeting not only improves the user experience but also significantly enhances the efficiency of advertising spend.
Real-time bidding (RTB) strategies for ad placement
Real-Time Bidding (RTB) has transformed the way digital ad space is bought and sold. This automated process allows advertisers to bid on individual ad impressions in real-time, based on the user's profile and the context of the webpage. RTB enables highly targeted ad placements by considering factors such as the user's location, device type, and browsing behavior.
The speed and precision of RTB make it an invaluable tool for targeted advertising. In the milliseconds it takes for a webpage to load, RTB platforms can evaluate thousands of bids and select the most relevant ad for that specific user. This ensures that advertisers reach their desired audience at the right moment, maximizing the impact of their campaigns and driving higher engagement rates.
Dynamic creative optimization (DCO) in targeted campaigns
Dynamic Creative Optimization (DCO) takes personalization to the next level by automatically adjusting ad content based on user data and context. This technology allows advertisers to create multiple versions of an ad and dynamically serve the most appropriate version to each user. DCO can customize elements such as images, text, calls-to-action, and even product recommendations in real-time.
For instance, a clothing retailer using DCO might show different product images based on the user's browsing history, local weather conditions, or past purchase behavior. This level of customization ensures that each ad is as relevant and compelling as possible, significantly increasing the chances of engagement and conversion.
Lookalike audience modeling for expanded reach
Lookalike audience modeling is a powerful technique that allows advertisers to expand their reach by targeting users who share similar characteristics with their existing customers. By analyzing the profiles of their most valuable customers, businesses can identify common traits and behaviors, and then use this information to find new prospects with similar attributes.
This approach is particularly effective for businesses looking to grow their customer base while maintaining high-quality leads. For example, a subscription-based service might use lookalike modeling to target users who exhibit similar online behaviors to their most loyal subscribers. This targeted approach often results in higher conversion rates and more efficient use of advertising budgets.
Cross-channel retargeting strategies
Cross-channel retargeting is a sophisticated approach that allows advertisers to reach potential customers across multiple platforms and devices. By maintaining a consistent message and brand presence across various touchpoints, businesses can reinforce their marketing message and increase the likelihood of conversion.
Pixel-based vs. list-based retargeting methods
Retargeting methods can be broadly categorized into pixel-based and list-based approaches. Pixel-based retargeting uses a small piece of code (pixel) placed on a website to track user behavior and serve ads accordingly. This method is highly effective for reaching users who have recently interacted with a website but haven't made a purchase.
List-based retargeting, on the other hand, relies on customer data provided by the advertiser, such as email lists or CRM data. This approach allows for more precise targeting based on known customer information but may have a more limited reach compared to pixel-based methods. Many successful retargeting campaigns combine both approaches to maximize their effectiveness and reach.
Sequential retargeting across social media platforms
Sequential retargeting is an advanced technique that delivers a series of ads to users in a specific order across different social media platforms. This approach allows advertisers to tell a cohesive story or guide users through the sales funnel by presenting increasingly targeted messages as the user progresses through their decision-making process.
For example, a travel company might start with awareness-building ads on Facebook, followed by more detailed destination information on Instagram, and finally, exclusive deal offers on Twitter. By orchestrating this sequential journey across platforms, advertisers can create a more engaging and persuasive narrative that drives users towards conversion.
Integrating CRM data for enhanced retargeting precision
Integrating Customer Relationship Management (CRM) data into retargeting strategies can significantly enhance targeting precision. By combining offline customer data with online behavior insights, businesses can create highly personalized ad experiences that speak directly to individual customer needs and preferences.
For instance, a retailer might use CRM data to identify customers who have made in-store purchases but haven't engaged with the brand online. They could then create targeted online ads to encourage these customers to explore the brand's e-commerce offerings. This integration of online and offline data allows for a more holistic approach to customer engagement and can lead to increased loyalty and lifetime value.
Measuring and optimizing ad engagement metrics
To ensure the success of targeted advertising campaigns, it's crucial to continuously measure and optimize various engagement metrics. By closely monitoring these key performance indicators, advertisers can refine their strategies and maximize the impact of their marketing efforts.
Click-through rate (CTR) analysis and improvement techniques
Click-Through Rate (CTR) is a fundamental metric that measures the percentage of users who click on an ad after viewing it. A high CTR indicates that the ad is relevant and compelling to the target audience. To improve CTR, advertisers should focus on creating eye-catching visuals, crafting compelling ad copy, and ensuring that the ad is highly relevant to the target audience.
One effective technique for improving CTR is A/B testing, where multiple versions of an ad are created and tested to determine which performs best. By systematically testing different elements such as headlines, images, and calls-to-action, advertisers can identify the most effective combinations and continuously refine their ads for maximum impact.
Conversion rate optimization (CRO) for targeted ads
While a high CTR is important, the ultimate goal of most advertising campaigns is to drive conversions. Conversion Rate Optimization (CRO) focuses on improving the percentage of users who take a desired action after clicking on an ad, such as making a purchase or signing up for a newsletter.
To optimize conversion rates, advertisers should ensure that the landing page experience is seamless and aligned with the ad's message. This includes creating clear and compelling calls-to-action, optimizing page load times, and providing relevant and valuable content. Additionally, implementing trust signals such as customer testimonials or security badges can help alleviate potential concerns and encourage conversions.
Attribution modeling for multi-touch customer journeys
In today's complex digital landscape, customers often interact with multiple touchpoints before making a purchase decision. Attribution modeling helps advertisers understand the impact of each touchpoint in the customer journey and allocate credit appropriately. This insight is crucial for optimizing marketing spend and determining which channels are most effective at driving conversions.
There are various attribution models available, ranging from simple last-click models to more sophisticated multi-touch attribution approaches. Advanced attribution models use machine learning algorithms to analyze vast amounts of data and provide a more nuanced understanding of the customer journey. By leveraging these insights, advertisers can make more informed decisions about budget allocation and campaign optimization.
Privacy-compliant targeting in the Post-GDPR era
As data privacy regulations such as the General Data Protection Regulation (GDPR) have come into effect, advertisers must adapt their targeting strategies to ensure compliance while still delivering effective, personalized ads. This new landscape has led to the development of innovative approaches that balance privacy concerns with the need for targeted advertising.
Contextual targeting as a cookie-less alternative
Contextual targeting has gained renewed importance as a privacy-friendly alternative to cookie-based targeting. Instead of relying on user data, contextual targeting focuses on the content of the webpage where the ad is displayed. By analyzing factors such as keywords, topics, and sentiment, advertisers can place ads in contexts that are likely to be relevant to the user's current interests.
For example, an ad for running shoes might be displayed on a fitness blog or a news article about marathon training. This approach ensures that ads remain relevant without relying on personal data, making it an attractive option in the post-GDPR era. Advanced contextual targeting technologies use natural language processing and machine learning to provide increasingly sophisticated and accurate content analysis.
First-party data strategies for personalized advertising
As third-party cookies become less reliable, many advertisers are turning to first-party data strategies to power their personalized advertising efforts. First-party data is information collected directly from customers through interactions with a company's website, app, or other owned channels. This data is typically more accurate and privacy-compliant than third-party data.
To leverage first-party data effectively, businesses need to implement robust data collection and management systems. This might include implementing customer data platforms (CDPs) to unify data from various sources and create comprehensive customer profiles. By using this owned data to inform targeting decisions, advertisers can create highly personalized experiences while respecting user privacy preferences.
Implementing consent management platforms (CMPs)
Consent Management Platforms (CMPs) have become essential tools for advertisers operating in regions with strict privacy regulations. These platforms allow websites to obtain and manage user consent for data collection and ad targeting. By providing users with clear information about how their data will be used and giving them control over their privacy settings, CMPs help build trust and ensure compliance with regulations like GDPR.
Implementing a CMP not only helps with legal compliance but can also improve the overall user experience. When users feel in control of their data and see that their privacy preferences are respected, they may be more likely to engage with personalized content and ads. This transparency can lead to more positive brand perceptions and potentially higher engagement rates.
Emerging technologies in targeted advertising
The field of targeted advertising is constantly evolving, with new technologies emerging that promise to revolutionize how businesses connect with their audiences. These innovations are opening up exciting possibilities for more precise, engaging, and effective advertising campaigns.
AI-powered predictive analytics for customer behavior
Artificial Intelligence (AI) is transforming predictive analytics in targeted advertising. Advanced AI algorithms can process vast amounts of data to identify complex patterns and predict future customer behaviors with unprecedented accuracy. This capability allows advertisers to anticipate customer needs and preferences, enabling them to serve highly relevant ads at the optimal moment.
For instance, AI-powered systems might analyze a combination of browsing history, purchase patterns, and even external factors like weather or economic indicators to predict when a customer is most likely to make a particular type of purchase. This level of insight allows for incredibly precise targeting and timing of ad delivery, significantly increasing the chances of engagement and conversion.
Voice search optimization for smart device targeting
With the rising popularity of smart speakers and voice-activated devices, voice search optimization has become a critical consideration for targeted advertising. As more consumers use voice commands to search for information and make purchases, advertisers need to adapt their strategies to capture this growing market.
Voice search optimization involves understanding the unique characteristics of spoken queries, which tend to be longer and more conversational than typed searches. Advertisers need to optimize their content and keywords for natural language patterns and focus on providing concise, direct answers to common questions. Additionally, location-based targeting becomes even more crucial in voice search, as many voice queries are related to local information or services.
Augmented reality (AR) in interactive targeted ads
Augmented Reality (AR) is opening up new frontiers in interactive advertising. By overlaying digital content onto the real world, AR allows advertisers to create immersive, engaging experiences that blur the line between physical and digital realms. This technology is particularly effective for products that benefit from visualization or try-before-you-buy experiences.
For example, a furniture retailer might use AR to allow customers to visualize how a piece of furniture would look in their home before making a purchase. Cosmetics brands can offer virtual try-on experiences, allowing users to see how different products would look on their own face. These interactive AR ads not only capture attention but also provide valuable utility to consumers, increasing engagement and driving conversions.
As these emerging technologies continue to evolve and mature, they will undoubtedly shape the future of targeted advertising. By staying at the forefront of these innovations, advertisers can create more compelling, personalized, and effective campaigns that resonate with their audience and drive business growth.