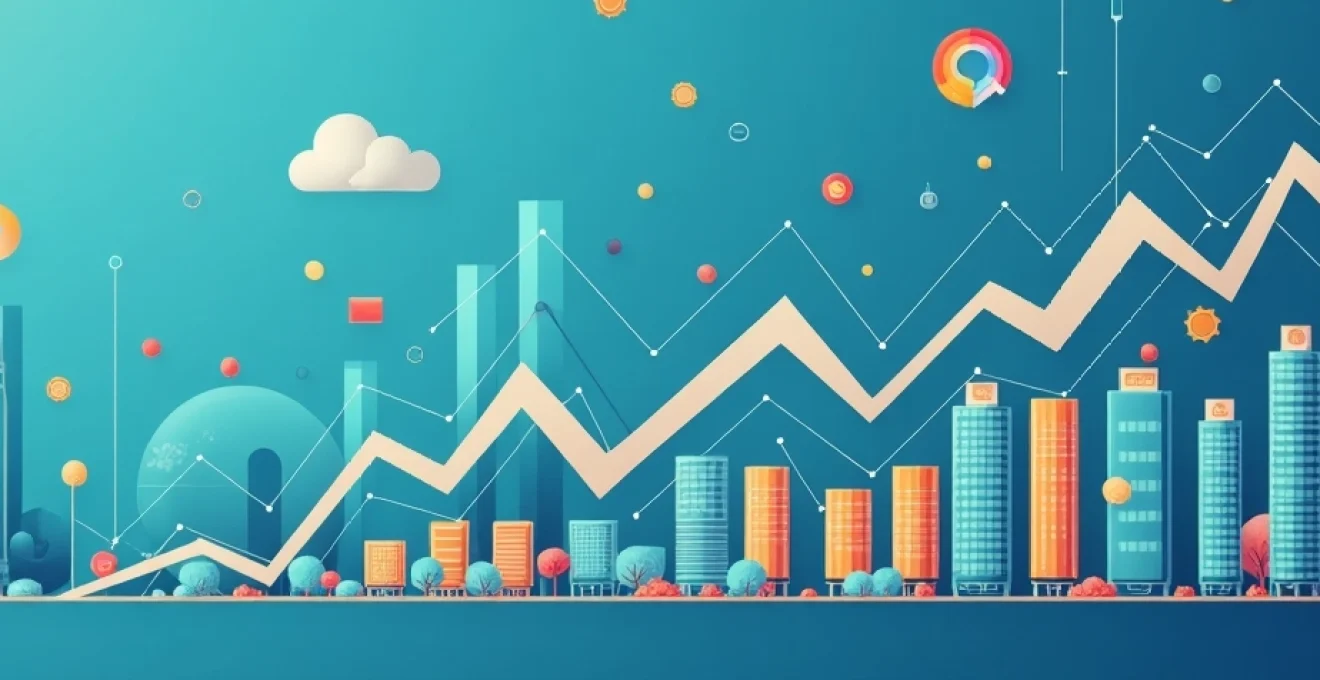
In today's data-driven marketing landscape, understanding customer behavior is crucial for businesses aiming to create personalized and effective marketing strategies. Behavioral analysis has emerged as a powerful tool, providing deep insights into customer preferences, habits, and decision-making processes. By leveraging these insights, companies can tailor their marketing efforts to resonate with specific customer segments, ultimately driving engagement and conversions.
Behavioral analysis goes beyond traditional demographic segmentation, delving into the psychological and emotional factors that influence consumer choices. This approach allows marketers to create highly targeted campaigns that speak directly to the needs and desires of their audience. As technology continues to evolve, the methods for collecting and analyzing behavioral data have become increasingly sophisticated, offering unprecedented opportunities for businesses to connect with their customers on a more meaningful level.
Fundamentals of behavioral analysis in customer profiling
At its core, behavioral analysis in customer profiling involves the systematic examination of customer actions, interactions, and preferences across various touchpoints. This process aims to create a comprehensive picture of each customer's journey, from initial awareness to post-purchase behavior. By understanding these patterns, businesses can identify key drivers of customer decisions and tailor their marketing strategies accordingly.
One of the fundamental aspects of behavioral analysis is the creation of detailed customer profiles. These profiles go beyond basic demographic information, incorporating data on purchasing history, browsing behavior, social media interactions, and customer service inquiries. The result is a multidimensional view of each customer, allowing for more nuanced and effective targeting.
Another critical component of behavioral analysis is the identification of customer segments based on shared behavioral traits. This segmentation allows marketers to create targeted campaigns that resonate with specific groups, rather than relying on a one-size-fits-all approach. For example, a segment of price-sensitive customers might respond well to discount offers, while another segment of luxury-oriented customers might be more interested in exclusive, premium products.
The power of behavioral analysis lies in its ability to reveal hidden patterns and correlations that might not be immediately apparent. By applying advanced analytics techniques, businesses can uncover valuable insights that drive more informed decision-making across all aspects of their marketing strategy.
Data collection methodologies for customer behavior tracking
Effective behavioral analysis relies on robust data collection methods that capture a wide range of customer interactions. As technology has advanced, the tools and techniques available for tracking customer behavior have become increasingly sophisticated, allowing for more comprehensive and accurate data collection.
Web analytics tools: Google Analytics vs. Adobe Analytics
Web analytics tools play a crucial role in tracking customer behavior online. Two of the most popular platforms in this space are Google Analytics and Adobe Analytics. Google Analytics, known for its user-friendly interface and extensive integration capabilities, provides a wealth of data on website traffic, user engagement, and conversion rates. It offers features such as real-time reporting, custom event tracking, and advanced segmentation options.
On the other hand, Adobe Analytics is favored by many enterprise-level organizations for its robust data processing capabilities and advanced attribution modeling. It excels in handling large volumes of data and provides more granular control over data collection and analysis. Both tools offer valuable insights into customer behavior, with the choice often depending on the specific needs and scale of the business.
Customer relationship management (CRM) systems integration
CRM systems serve as a central repository for customer data, making them an invaluable resource for behavioral analysis. By integrating CRM data with other data sources, businesses can create a more complete picture of their customers' behavior across various touchpoints. This integration allows for the tracking of long-term customer relationships, including purchase history, customer service interactions, and engagement with marketing campaigns.
Modern CRM systems often come with built-in analytics capabilities, allowing businesses to segment customers based on behavioral data and automate personalized marketing efforts. The key to effective CRM integration lies in ensuring data quality and consistency across all touchpoints, enabling accurate and actionable insights.
Social media listening platforms: sprout social and hootsuite insights
Social media has become a rich source of behavioral data, offering insights into customer preferences, sentiments, and brand perceptions. Social media listening platforms like Sprout Social and Hootsuite Insights allow businesses to monitor and analyze social media conversations at scale. These tools can track mentions of a brand, competitors, or specific topics, providing valuable context for customer behavior.
Sprout Social offers features such as sentiment analysis, trend identification, and competitor comparisons, helping businesses understand how their brand is perceived in the social media landscape. Hootsuite Insights focuses on real-time social media monitoring and provides in-depth analytics on audience demographics and engagement patterns. By leveraging these platforms, marketers can gain a deeper understanding of their customers' social media behavior and tailor their strategies accordingly.
Mobile app usage tracking: firebase analytics and mixpanel
With the increasing prevalence of mobile devices, tracking customer behavior within mobile apps has become essential for many businesses. Firebase Analytics, a Google product, offers a comprehensive suite of app analytics tools, including user engagement metrics, crash reporting, and A/B testing capabilities. It provides detailed insights into user behavior within the app, helping developers and marketers optimize the user experience and drive engagement.
Mixpanel, another popular mobile analytics platform, focuses on event-based tracking and user segmentation. It allows businesses to create custom event funnels and cohort analyses, providing granular insights into how users interact with specific features of an app. By leveraging these mobile analytics tools, businesses can gain a deeper understanding of their customers' mobile behavior and optimize their app strategies accordingly.
Machine learning algorithms in behavioral pattern recognition
The application of machine learning algorithms has revolutionized the field of behavioral pattern recognition, enabling businesses to uncover complex patterns and predict future customer behavior with unprecedented accuracy. These algorithms can process vast amounts of data, identifying correlations and trends that would be impossible for humans to detect manually.
Clustering techniques: K-means vs. hierarchical clustering
Clustering algorithms are essential tools for segmenting customers based on their behavioral patterns. Two popular clustering techniques are K-means and hierarchical clustering. K-means clustering is an iterative algorithm that groups data points into a predetermined number of clusters based on their similarity. It's particularly useful for large datasets and can quickly identify distinct customer segments based on behavioral traits.
Hierarchical clustering, on the other hand, creates a tree-like structure of clusters, allowing for a more nuanced understanding of the relationships between different customer groups. This technique is valuable when the number of clusters is not known in advance and when exploring the hierarchical structure of customer segments is important.
Predictive modeling: random forests and gradient boosting machines
Predictive modeling techniques such as Random Forests and Gradient Boosting Machines are powerful tools for forecasting customer behavior. Random Forests, an ensemble learning method, combines multiple decision trees to create robust predictions. It's particularly effective at handling complex datasets with many variables and can provide insights into the relative importance of different behavioral factors.
Gradient Boosting Machines, including popular implementations like XGBoost, build predictive models by iteratively combining weak learners into a strong predictor. These algorithms excel at capturing non-linear relationships in data and are often used for tasks such as predicting customer churn or estimating lifetime value.
Natural Language Processing for sentiment analysis
Natural Language Processing (NLP) techniques have become increasingly important in behavioral analysis, particularly for understanding customer sentiment and preferences expressed in text form. Sentiment analysis algorithms can automatically classify the emotional tone of customer reviews, social media posts, and support tickets, providing valuable insights into customer attitudes and experiences.
Advanced NLP models, such as those based on deep learning architectures like BERT (Bidirectional Encoder Representations from Transformers), can capture nuanced contextual information in text data. This allows for more accurate sentiment analysis and can even detect subtle shifts in customer opinions over time.
Deep learning approaches: Recurrent Neural Networks for sequence prediction
Recurrent Neural Networks (RNNs) and their variants, such as Long Short-Term Memory (LSTM) networks, are particularly well-suited for analyzing sequential behavioral data. These deep learning models can capture temporal dependencies in customer behavior, making them valuable for tasks such as predicting the next likely action in a customer journey or forecasting future purchasing patterns.
RNNs can process sequences of varying lengths, making them ideal for analyzing customer interactions that unfold over time, such as browsing sessions or multi-step purchasing processes. By leveraging these advanced deep learning techniques, businesses can gain a more nuanced understanding of the temporal aspects of customer behavior, enabling more accurate predictions and personalized marketing strategies.
Psychographic segmentation techniques for targeted marketing
Psychographic segmentation goes beyond traditional demographic segmentation by focusing on customers' psychological attributes, values, and lifestyles. This approach allows marketers to create more nuanced and effective targeting strategies that resonate with customers on a deeper level.
VALS framework and lifestyle segmentation
The Values and Lifestyles (VALS) framework is a widely used psychographic segmentation tool that categorizes individuals based on their primary motivations and resources. Developed by SRI International, VALS divides consumers into eight distinct groups, each with its own set of values, attitudes, and purchasing behaviors. These groups range from "Innovators," who are sophisticated and open to new ideas, to "Survivors," who focus on meeting basic needs and security.
By understanding which VALS segments their customers belong to, businesses can tailor their marketing messages and product offerings to align with the values and motivations of each group. For example, a luxury brand might focus on appealing to the status-conscious "Achievers" segment, while a budget-friendly retailer might target the practical "Believers" segment.
Personality-based targeting: the Big Five model application
The Big Five personality model, also known as the Five-Factor Model (FFM), is a widely accepted framework for understanding human personality. The five traits - Openness, Conscientiousness, Extraversion, Agreeableness, and Neuroticism (OCEAN) - provide a comprehensive view of an individual's personality and can be used to inform targeted marketing strategies.
By analyzing customer behavior and language use, businesses can infer personality traits and tailor their marketing approaches accordingly. For instance, individuals high in openness might be more receptive to innovative products and creative marketing campaigns, while those high in conscientiousness might respond better to messages emphasizing reliability and efficiency.
Behavioral economics principles in marketing strategies
Behavioral economics, which combines insights from psychology and economics, offers valuable principles for understanding and influencing consumer behavior. Concepts such as loss aversion, anchoring, and the endowment effect can be applied to marketing strategies to enhance their effectiveness.
For example, the principle of scarcity can be leveraged to create a sense of urgency in marketing messages, encouraging customers to act quickly. Similarly, the concept of social proof can be used to highlight popular products or services, influencing customer decisions through the power of collective behavior.
Understanding and applying behavioral economics principles allows marketers to design more persuasive and effective campaigns that align with the natural decision-making processes of their target audience.
Real-time personalization engines and dynamic content delivery
Real-time personalization engines represent the cutting edge of targeted marketing, allowing businesses to deliver highly relevant content and offers to customers in the moment. These systems leverage behavioral data, machine learning algorithms, and real-time decision-making capabilities to create personalized experiences across various touchpoints.
Dynamic content delivery systems can adapt website content, email marketing messages, and even in-app experiences based on a user's behavior and preferences. For example, an e-commerce site might dynamically adjust product recommendations based on a user's browsing history, recent purchases, and current session behavior.
The key to effective real-time personalization lies in the ability to process and act on data quickly. This requires robust data infrastructure, advanced analytics capabilities, and seamless integration across various marketing channels. When implemented successfully, real-time personalization can significantly enhance customer engagement, conversion rates, and overall customer satisfaction.
Ethical considerations and data privacy in behavioral analysis
As behavioral analysis becomes increasingly sophisticated, it's crucial for businesses to consider the ethical implications of their data collection and usage practices. Balancing the desire for deep customer insights with the need to protect individual privacy is a complex challenge that requires careful consideration and robust safeguards.
GDPR compliance in customer data collection and usage
The General Data Protection Regulation (GDPR) has set a new standard for data privacy and protection, particularly in the European Union. Compliance with GDPR requires businesses to be transparent about their data collection practices, obtain explicit consent from users, and provide mechanisms for users to access, correct, and delete their personal data.
For behavioral analysis, GDPR compliance means ensuring that all data collection and processing activities are lawful, fair, and transparent. This includes implementing data minimization practices, ensuring data security, and conducting data protection impact assessments when necessary.
Transparency in AI-driven decision making for marketing
As artificial intelligence plays an increasingly significant role in marketing decision-making, ensuring transparency in these processes becomes crucial. Customers should be informed when AI systems are being used to make decisions that affect them, and businesses should be able to explain the logic behind these decisions.
Transparency also extends to the use of behavioral data in targeting and personalization efforts. Customers should have a clear understanding of how their data is being used to tailor their experiences and should be given control over these personalization features.
Balancing personalization and privacy: opt-in strategies
Implementing robust opt-in strategies is essential for maintaining a balance between personalization and privacy. Rather than assuming consent, businesses should provide clear, easily understandable options for customers to choose what data they're willing to share and how it can be used.
Granular opt-in options allow customers to have more control over their data, potentially increasing trust and willingness to engage with personalized experiences. For example, a customer might choose to share browsing data for product recommendations but opt out of location tracking for targeted ads.
By prioritizing ethical data practices and respecting customer privacy, businesses can build trust and foster long-term relationships with their customers, ultimately creating more sustainable and effective behavioral analysis strategies.
As behavioral analysis continues to evolve, it will undoubtedly play an increasingly central role in shaping marketing strategies and customer experiences. By leveraging advanced data collection methodologies, sophisticated machine learning algorithms, and real-time personalization engines, businesses can gain unprecedented insights into customer behavior and preferences. However, it's crucial to approach these powerful tools with a strong ethical framework, ensuring that customer privacy and trust remain at the forefront of all behavioral analysis efforts.